
PCA dimensions are also called axes or Factors. It is a projection method as it projects observations from a p-dimensional space with p variables to a k-dimensional space (where k < p) so as to conserve the maximum amount of information (information is measured here through the total variance of the dataset) from the initial dimensions. Principal Component Analysis is one of the most frequently used multivariate data analysis methods for dimensionality reduction.
Xlstat principal component analysis how to#
We also provide many free learning resources on the web, such as a tutorial on how to run PCA in XLSTAT as well as a guide to choose the best data mining or multivariate data analysis method according to your situation. Launch a pre-configured Agglomerative Hierarchical Clustering (AHC) using the dendrogram button below the factor scores table.Copy your PCA coordinates from the results report to use them in further analyses.Customize your correlation circle, your observations plot or your biplots as standard Excel charts.Filter out variables or observations according to different criteria to optimize PCA map readability.Add supplementary variables or observations.Run your PCA on raw data or on dissimilarity matrices.
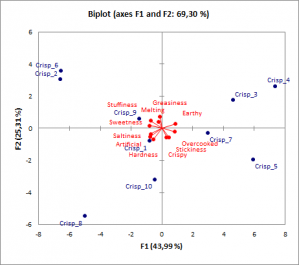
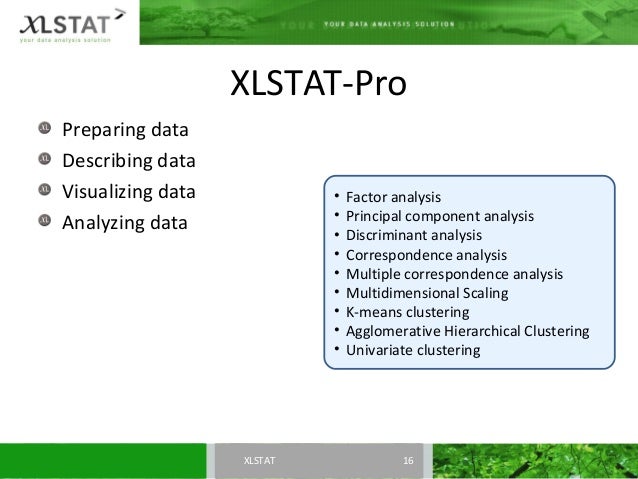
XLSTAT proposes several standard and advanced options that will let you gain a deep insight into your data: XLSTAT provides a complete and flexible PCA feature to explore your data directly in Excel. It is widely used in biostatistics, marketing, sociology, and many other fields. Principal Component Analysis ( PCA) is a powerful and popular multivariate analysis method that lets you investigate multidimensional datasets with quantitative variables.
